Friday, 26th of January 2024 at 11:00 am – 12:30 pm
Chairs: Sabine Baumgart & Axel Schulz
News from… Berlin (BIH) by Leona Simon
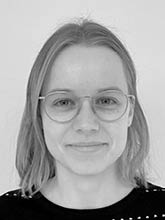
Institute of Pathology, Charité—Universitätsmedizin Berlin and University of Potsdam
Getting Patient-Derived Organoid Samples ready for Mass Cytometry
Patient-derived organoids (PDOs) are a model of choice to study inter- and intratumoral cell heterogeneity. To understand resistance mechanisms and therapy responses in cancer, single cell analysis is key. Formaldehyde (FA) fixation before single cell dissociation has been proposed to prevent changes in the signaling state caused by stress responses as a result of digestion. When dissociating FA-fixed PDOs, including human colon organoids originating from tumor or normal tissue, however, it was not possible to obtain a single-cell solution suitable for subsequent Mass Cytometry (CyTOF) analysis.
Here, we propose to include an additional Antigen Retrieval (AGR) step in the dissociation protocol, which renders chemically fixed cell-cell adhesion molecules more accessible for proteolytic cleavage, thereby facilitating the dissociation process.
We were able to show that including AGR in the organoid dissociation protocol significantly improves the dissociation efficiency of PDOs, making them suitable for downstream Mass Cytometry analysis. It was the first time that we could efficiently obtain a single-cell suspension from PDOs derived from normal colon tissue. Additionally, AGR also improves signal intensities of many markers measured by CyTOF. This offers opportunities to investigate the early stages of cancer and gain deeper insights into the molecular mechanisms influencing different therapy responses.
News from… UIm by Kathrin Niedermayer

Department of Obstetrics and Gynecology, Ulm University, Ulm, Germa
Mass cytometric detection of DNA repair proficiency in circulating tumor cells to predict chemoresistance of metastatic breast cancer patients
Circulating tumor cells (CTCs) are shed from solid tumors and can be analyzed by a blood-based liquid biopsy to gain insight into treatment responses and metastatic recurrence. Due to their rarity of 1-10 CTCs/7.5 ml whole blood from metastatic breast cancer (mBC) patients, the analysis of CTCs is challenging and commonly based on immunomagnetic technologies using antibodies against EpCAM, led by the FDA-approved CellSearch system. This study used mass cytometry for the identification and characterization of CTCs from eleven mBC patients. Functional analysis focused on DNA damage responses, particularly the DNA repair pathway of homologous recombination.
Blood from mBC patients was collected in a CellSave and two EDTA tubes per blood draw. CellSave tubes were used for identification of CTCs using the CellSearch technology. EDTA tubes were used to isolate plasma and CTCs together with peripheral blood mononuclear cells (PBMCs). Cell free DNA (cfDNA) was isolated from the plasma samples for further analysis regarding tumor fraction and homologous recombination deficiency (HRD). CTC/PBMC mixtures were phenotyped by mass cytometry using a panel of 13 antibodies such as directed against γH2AX and RAD51.
Mass cytometry allowed the identification of CTCs with a sensitivity of 95% compared to the CellSearch technology. The homologous recombination status in CTCs was established with a concordance of 73% compared with an HRD score determined via shallow genome sequencing of cfDNA. In conclusion, our mass cytometric marker set enables identification of CTCs in blood samples from mBC patients and assessment of their proficiency to perform homologous recombination.
News from… Jena by Reza Gheitasi
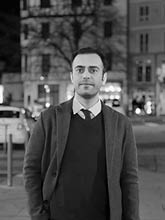
Institute for Infectious Diseases and Infection Control, Jena University Hospital, – Friedrich Schiller University Jena, Germany.
Age- and Sex-Associated Changes in Immune Cell Populations
It is already known that the function of our immune system becomes impaired during aging process. Besides the age, also sex plays a significant role on the incidence and severity of infectious diseases, but barely is known about the extent of age-associated changes between men and women.
In this study, we investigated the age and sex interaction changes in the immune cell composition of 243 (120 females, 123 males) inhabitants between the age of 19 and 93 from Neustadt am Rennsteig in Thuringia, as part of the CoNAN-Study. Peripheral blood was processed using the MAXPAR Deep Immune Profiling Assay, measured on a Helios instrument, and analyzed with Pathsetter software v3 (Standard BioTools, South San Francisco, CA, USA). Unlike the other published studies, we further determined changes of either frequency or absolute counts of major leukocyte subsets by using smoothing spline regression and therefore have circumvented to group the cohort into age ranges.
Our data confirm previous findings such as the decrease in the number of naïve lymphocytes, including naïve T cells and naïve B cells, during aging. However, this decline was particularly observed in men, while women retain their naïve B cells one decade longer than men. Since naïve lymphocytes are important for mounting an immune response to new antigens, an age-associated decline might significantly impact on the cellular and humoral immunity in context of new infections. Additionally, an age-associated increase in effector memory T cells (particularly central memory CD8+ T cells) was observed in men, which was aligned with a decreasing trend in dendritic cell counts in both men and women. In the context of innate immune cells, an increase of transitional monocytes occurred specific to men, while other innate immune cells such as granulocytes (Neus, Baso, Eos) did not show any compositional changes over the lifespan.
Using multi-dimensional mass spectrometry combined with the spline regression approach uncovers new insights into age-sex-interaction-specific changes in immune cell composition that occur with aging. These findings may help to understand the immune status prevalent among various age cohorts. Nevertheless, it is necessary to extend the analysis of other cohorts for validation of our findings since our study was restricted to one village in rural Thuringia only.
News from… Berlin (DRFZ) by Lena Teichert
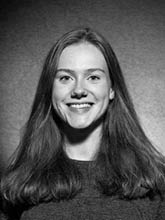
Deutsches Rheuma Forschungszentrum Berlin, a Leibniz Institut, Berlin, Germany
Department of Rheumatology and Clinical Immunology, Charité – Universitätsmedizin Berlin, Freie Universität Berlin and Humboldt-Universität zu Berlin, Berlin, Germany
Mass cytometry analysis of B cell subsets in IgG4-related disease
IgG4-related disease (IgG4-RD) is a rare chronic inflammatory condition of unknown etiology, characterized by an abundance of IgG4+ plasma cells, storiform fibrosis and obliterative phlebitis at affected sites, and usually manageable with glucocorticoids and B cell depleting therapies. It is well established that CD4+ cytotoxic T lymphocytes and plasmablasts play a role in the pathophysiology, but there is limited data on B cell disturbances. We therefore carried out an in-depth phenotypic analysis of B cell subsets from peripheral blood with mass cytometry in 25 IgG4-RD patients compared to 35 healthy controls.
We identified 8 significantly increased B cell clusters in IgG4-RD, among them activated and proliferating B cell subsets, most of which displayed IgG4-subclass expression, and plasmablasts. In addition, we found a higher proportion of extrafollicular double-negative and switched memory CXCR5-CD19low plasma cell precursors. When correlated to clinical features, we found a greater proportion of significantly increased B cell subsets in patients with a more active disease, as determined by the IgG4-RD Responder Index (RI).
These data demonstrate a high heterogeneity of B cell subsets significantly increased in IgG4-RD. The data may help elucidating the pathogenesis of IgG4-RD, identify patients with potential refractory disease, and may lead to the development of new and potentially individualized treatment options in the future.
News from… Köln by Patrick Hölker
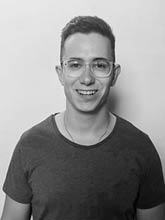
University of Cologne, Faculty of Medicine and University Hospital Cologne, Department I of Internal Medicine, Center for Integrated Oncology Aachen Bonn Cologne Duesseldorf, Cologne, Germany. Center for Molecular Medicine Cologne, University of Cologne, Cologne, Germany.
CECAD Center of Excellence on Cellular Stress Responses in Aging-Associated Diseases, University of Cologne, Cologne, Germany.
Advancing cancer research at the University of Cologne: The Impact of mass and and imaging mass cytometric technologies in hematological and solid cancers
In recent years, the integration of Cytometry by Time of Flight (CyTOF) technologies into biomedical research has notably increased. Its introduction in Cologne represents a significant advancement, with successful implementation across various departments. A key development is the adoption of Imaging Mass Cytometry (IMC) by numerous research groups. These groups actively decipher the complexities of disease processes, particularly in cancer research. The Department I of Internal Medicine in Cologne, focusing on studying and treating lymphoid malignancies, employs MC and IMC in researching aggressive lymphomas, leukemias, and their associated tumor immune microenvironments (CRC1530). This research, which includes analysis of both patient samples and murine cancer models, aims to enhance understanding of chronic lymphocytic leukemia, diffuse large B cell lymphoma, and mantle-cell lymphoma, aiding in the development of new treatment strategies. Furthermore, the application of MC and IMC contributes to understanding the interactions between malignant cells and their surrounding tumor immune microenvironment in various cancers. While the CyTOF initiatives primarily concentrate on hematological malignancies, they also include studies into solid tumors. Collaborations with the Institute of Pathology are integral to exploring the complexity of tumor microenvironmental interactions, especially in gastroesophageal adenocarcinoma, and examining the role of cancer-associated fibroblasts (CAFs) in salivary gland tumors. Our collaboration with CRC1399 enhances our analytical capabilities, especially in assessing the spatial heterogeneity of tumor cells and the composition of infiltrating immune cells in small-cell lung cancer (SCLC). In summary, the establishment of CyTOF single-cell analysis within the Cologne cancer research environment contributes significantly to the understanding of tumor microenvironmental interactions across a range of malignant diseases.
News from… Munic by Melissa Barroux
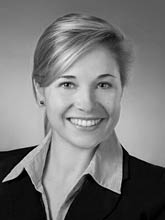
Evolution and Cancer Lab, Centre for Genomics and Computational Biology, Barts Cancer Institute, Queen Mary University of London, London, UK
Medical Clinic and Polyclinic II, Klinikum rechts der Isar, Technical University of Munich, München, Germany
Highly multiplexed imaging reveals immune cell dynamics correlating with treatment response in oesophageal adenocarcinoma
Locally advanced oesophageal adenocarcinoma (EAC) remains difficult to treat because of common resistance to neoadjuvant therapy and high recurrence rates. The role of the anti-tumor immune response remains incompletely understood.
EAC specimens were obtained from the prospective MEMORI trial. Patients were grouped into responders (REPs) or non-responders (NRPs) based on their response to neoadjuvant chemotherapy. Longitudinal imaging mass cytometry (IMC) was performed on 6 NRPs and 9 REPs at diagnosis, after the first cycle of chemotherapy and following resection. From single cell-level data, cell types were determined using clustering and hierarchical gating. IMC results were correlated with pathway analyses (RNA-Seq), neoantigen-profiling (whole exome sequencing) and clonal T-cell dynamics (T-cell receptor (TCR) sequencing).
We identified 28 cell clusters including tumor, stromal and immune cell subsets. NRPs showed a decrease in activated, cytotoxic and non-naïve CD8 T cells during treatment while REPs maintained CD8 T cell activation during therapy. The ratio between cytotoxic GranzymeB+ to PD-1+ CD4 and CD8 T cells was higher in REPs compared to NRPs at all timepoints, indicating a more effective T-cell phenotype in REPs. Consequently, neoantigen-profiling showed immuno-editing in REPs. Pathway enrichment analyses confirmed upregulated immune pathways in REPs and TCR analyses revealed clonal T-cell expansions in patients with good treatment response.
Longitudinal IMC and multi-omic analysis reveal T-cell dynamics in EAC, which correlate with treatment response. Our study highlights the quantitative and qualitative composition of the T-cell compartment as potential mediator of treatment response, suggesting combined immune-chemotherapy treatments as a potential efficacious approach for neoadjuvant treatment.